If you’re a user of social media site Twitter, you may have seen Lenny from the Simpsons appear a few times on your feed. If not Lenny, perhaps it was a Golden labrador, or maybe even US Senator, Mitch McConnell. Like most things these days, it all started off on Zoom. PhD student Colin Madland had discovered an issue highlighting the racial bias in the video-conferencing software Zoom, as he shared his findings on Twitter, he noticed something odd. When Colin posted an image of himself and a Black colleague, each time Twitter’s algorithm, in the preview image would fail to identify his Black colleague, and only show him. Coincidence maybe? Unfortunately not, as many users began testing Twitter’s algorithm on cropping images, it became evident the algorithm is biased to favour white men over Black men (human or cartoon) and even light brown dogs over black dogs. A few tested white men vs white women, however this result, although in favour of the men seem to be incognitant compared to skin tone. The feature in question was Twitter’s algorithm on cropping images. Have you ever noticed that when you upload an image to Twitter, they get cropped? Sometimes that crop is perfect, and sometimes it removes your head, well if you’re a white male, it appears the algorithm is in your favour for the perfect crop.
From reading replies to this issue one thing became evident, as people attempted to defend Twitter on this, and point out that well, the engineers who ‘tested and built this,’ did not code this to be racist, it was an oversight. This highlighted that people still do not understand the effects of unconscious bias on tech. It was also concerning that in Twitters statement, echoed by its engineers who were replying to some of these threads, that well, they did some testing and according to Twitter, “Our team did test for bias before shipping the model and did not find evidence of racial or gender bias in our testing.” Unfortunately, this isn’t the first time we have heard of these lines when it comes to algorithms and people of colour. From TikTok algorithms suppressing content from people of colour to Google Photos tagging Black people as unfavourable terms, countless times we see the same statements about how “we have tested this algorithm and it seemed fine.” Twitter has suggested that it will look to open source this element of the algorithm, allowing the community to work on it and also help eliminate bias. Thinking about the demographics most prevent in the open-source machine learning community will this solve the problem or just enhance it?
As the Twitter community began to investigate the algorithm using unofficial tests, there was a pattern. There was always one missing test group in the various test data. The testing data often included images of men, cartoon characters, Marvel superheroes and even dogs, but what would the results look like when tested on Black women? I decided to run my own experiment on Twitter using models from the makeup foundation line, Fenty Beauty. For my experiment, I decided to use the Fenty Beauty make up range to determine how biased the Twitter algorithm when addressing colourism. Fenty has categorised different shades of skin tone in 4 categories, with each shade numbered as below. It is also a useful range as the images are often taken with the same level of lighting and camera set up, so the data set should yield fair results.
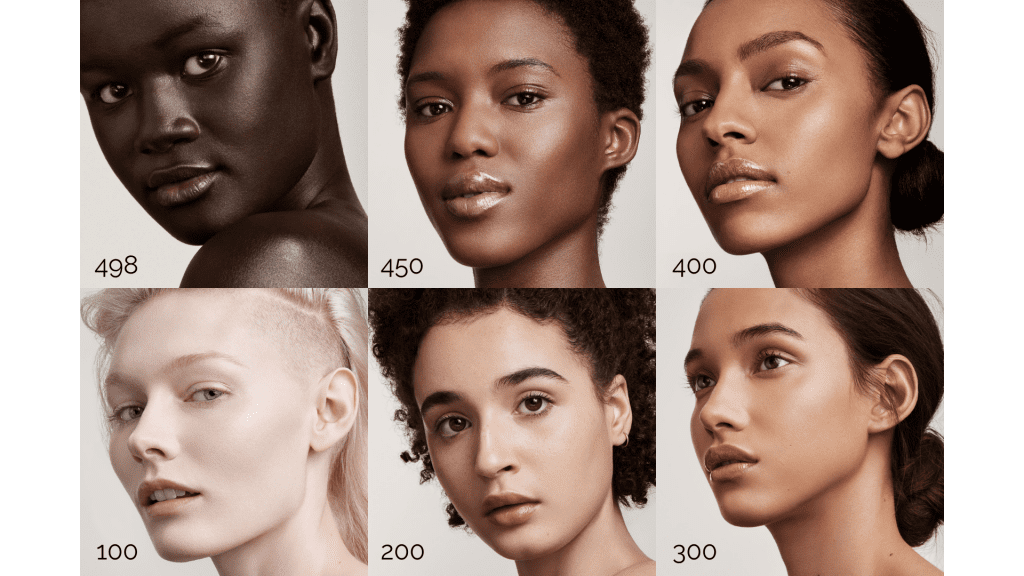
The shades were picked as the lightest shade of each group 100 to 400 with shade 450 and 498 added to give depth and texture. In each tweet, as you can see below, both images were placed on white backgrounds; size 4000px by 500px so as to distort the image length and allow the algorithm to detect a face. The Twitter algorithm, in theory, would analyse and then detect a face and crop it.
Of the seven tweets, three produced a clear favourite in cropping the lighter skin tone except for between shade 100 and 200, the darker skin tone was the crop focus, which is surprising considering shade 100 is of a white woman with straight hair.
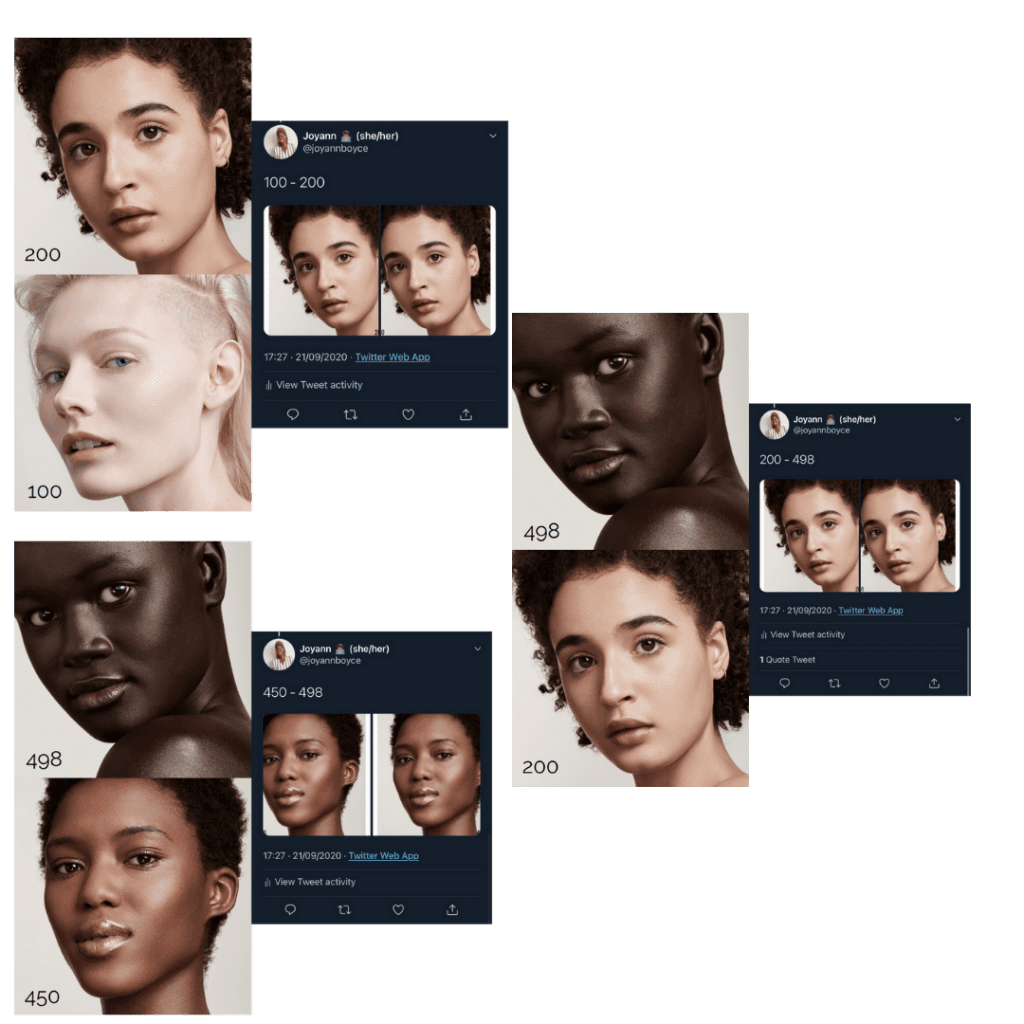
From the tweets which include Shade 300, it seems Twitter was not able to be detected a face all. This could be for many reasons:
- Of all the images, both eyes were not clearly visible, however this would question why was white space cropped in 50% of the tests using image 300. In theory if Shade 300 was not detected Twitter should have cropped to the other face/ shade in the images.
- Could it be possible that the Twitter algorithm is only able to pick up a face or skin tone difference 50% of the time when skin tone is mixed/ light brown.
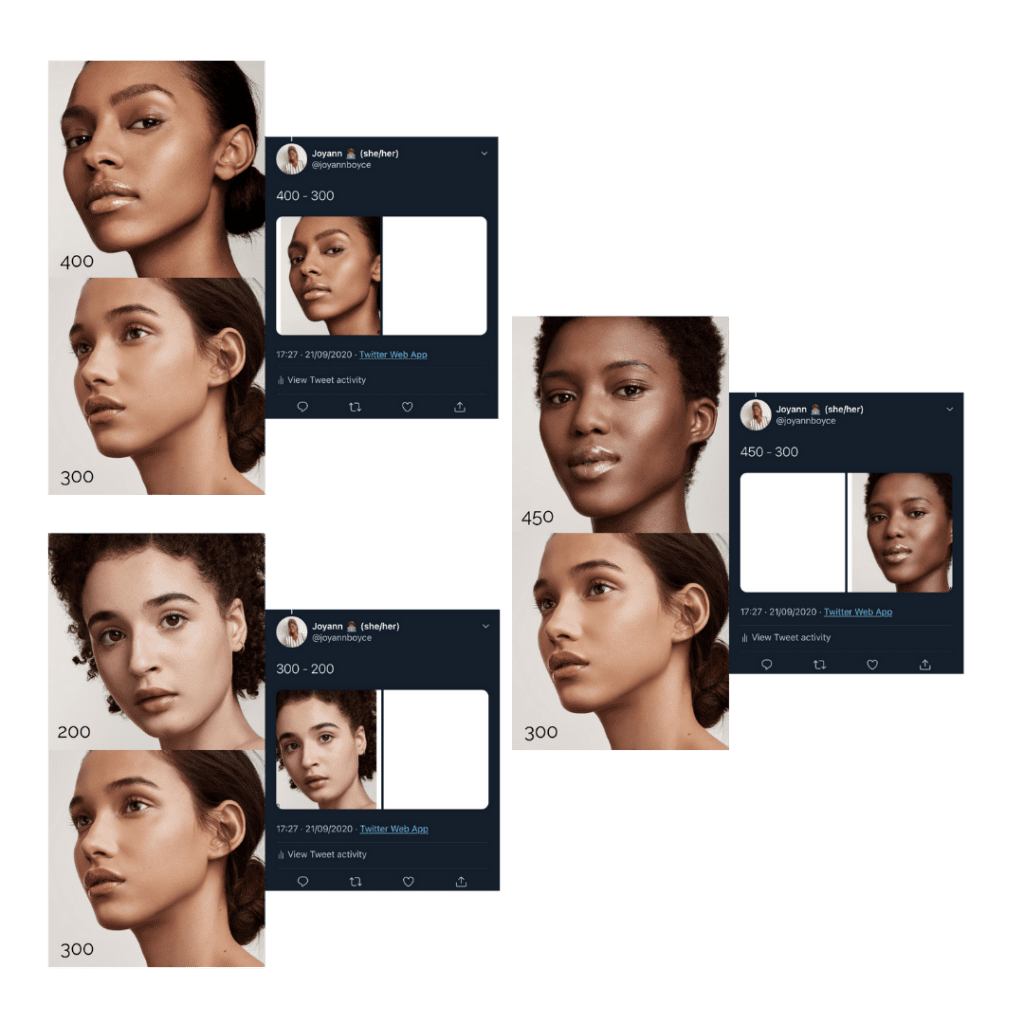
Of the results, when it came to Shade 450 and Shade 400, the Twitter algorithm was not able to detect their faces at all even though it was able to find the same faces in other tests. Could it be that when skintones are of close shades of brown the algorithm demand either to be of importance?
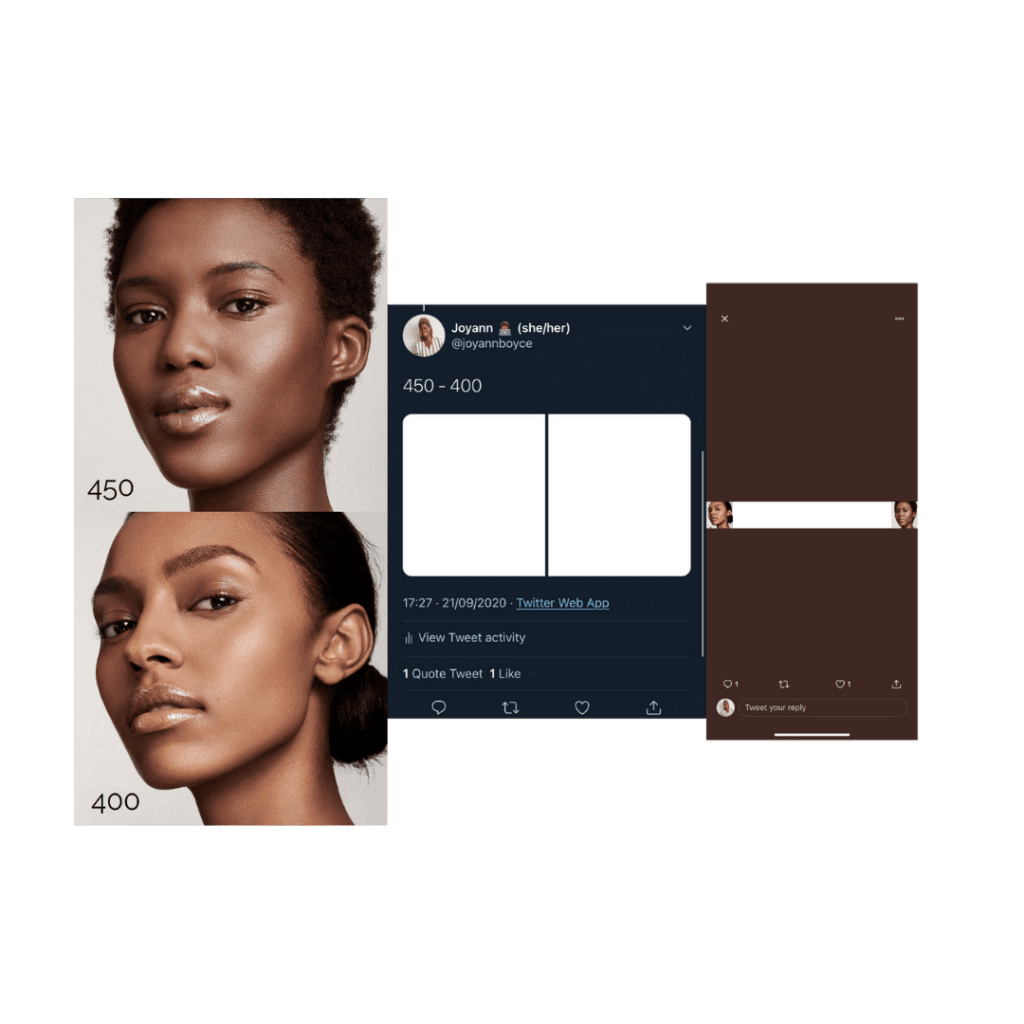
“Automated systems are not inherently neutral. They reflect priorities, preferences and prejudices – the coded gaze – of those who have the power to mould artificial intelligence.” This is a statement by the authors of the Gender Shades project, this statement is once again proven in the case of colourism and Twitters algorithm. The problem unfortunately doesn’t just lie at Twitter. As tech companies further rely on Artificial Intelligence and building machine learning models, the lack of diversity in their datasets and processes is being reflected in the tools and products they develop. Bias in machine models should be tested continuously, which is something Twitter clearly failed to do. In their response, they mentioned that during testing before deployment, they had not encountered any of the issues found by the community, but this seems so hard to believe and it would be interesting to see the dataset used to test. Another element observed from the community response was the lack of tests also focusing on colourism as often, most of these tests focused on a white male vs a black male. Colourism is prevalent in society and in some countries such as India, the caste system has long played a part in inequality in the country.
It is time for intersectionality in machine learning data sets. As we now see law enforcement and other areas of society start looking into AI and machine learning, unfortunately some of these institutions have been prevalent with systematic oppression and bias, and they will now be looking to use more systems which unfortunately will not be fit for purpose until intersectional diversity is at the forefront. Not only should companies be testing for race and sex bias, they should be testing for colourism as well. The inclusion of Black women should not be an afterthought after an algorithm is found to be biased. Tech needs to do better, companies need to do better.